L. Ding, A. E. Kuriyan, R. S. Ramchandran, C. C. Wykoff, and G. Sharma, “Weakly-supervised vessel detection in ultra-widefield fundus photography via iterative multi-modal registration and learning,” IEEE Trans. Medical Imaging, accepted for publication, to appear.
[Paper] [Supplementary] [Dataset] [GitHub] [Code Ocean capsule]
In this work, we focus on retinal vessel segmentation in ultra-widefield (UWF) fundus photography (FP), a retinal imaging modality that has received limited attention in prior works. UWF FP is noninvasive, only involves the capture of the retinal images under low-power illumination, and provides a wide field-of-view. We propose an annotation-efficient framework for vessel segmentation in UWF FP that eliminates the requirement of manually
labeled datasets for supervised learning.
- Contributions
- We present a novel iterative framework for vessel detection in UWF FP using DNNs. We rely on datasets that include concurrently captured UWF fluorescein angiography (FA) images, for which effective deep learning approaches for vessel detection have recently become available allowing for accurate vessel detection. The proposed framework
then jointly addresses precise registration between the vessel images for the modalities and vessel segmentation in UWF FP. - We construct a new ground truth labeled dataset, PRIME-FP20, to evaluate retinal vessel detection in UWF FP and to facilitate further work on this problem.
- The proposed framework provides a method for accurate vessel detection in UWF FP imagery, a modality that has received limited attention in prior works. The proposed approach significantly outperforms existing methods on the PRIME-FP20 dataset and, on narrow-field FP datasets, achieves performance comparable with state-of-the-art methods designed specifically for narrow-fiel FP. [GitHub] [Code Ocean capsule]
- We present a novel iterative framework for vessel detection in UWF FP using DNNs. We rely on datasets that include concurrently captured UWF fluorescein angiography (FA) images, for which effective deep learning approaches for vessel detection have recently become available allowing for accurate vessel detection. The proposed framework
- PRIME-FP20: Ultra-Widefield Fundus Photography Vessel Segmentation Dataset
- The PRIME-FP20 dataset contains 15 high-resolution ultra-widefield (UWF) fundus photography (FP), the corresponding labeled binary vessel maps, and the corresponding binary masks for the valid data region for the images. For each UWF FP image, a concurrently captured ultra-widefield fluorescein angiography (FA) is also included.
- UWF FP images are acquired using the Optos 200Tx camera (Optos plc, Dunfermline, United Kingdom). The system uses a scanning ophthamoscope with a low power laser
to capture dual red and green channel UWF FP images and a single channel FA image. - All images have the same resolution of 4000×4000 pixels and are stored as 8-bit TIFF format with lossless LZW compression.
- You can download the dataset from IEEE DataPort. This is an open-access dataset available to all IEEE users (IEEE Accounts are FREE).
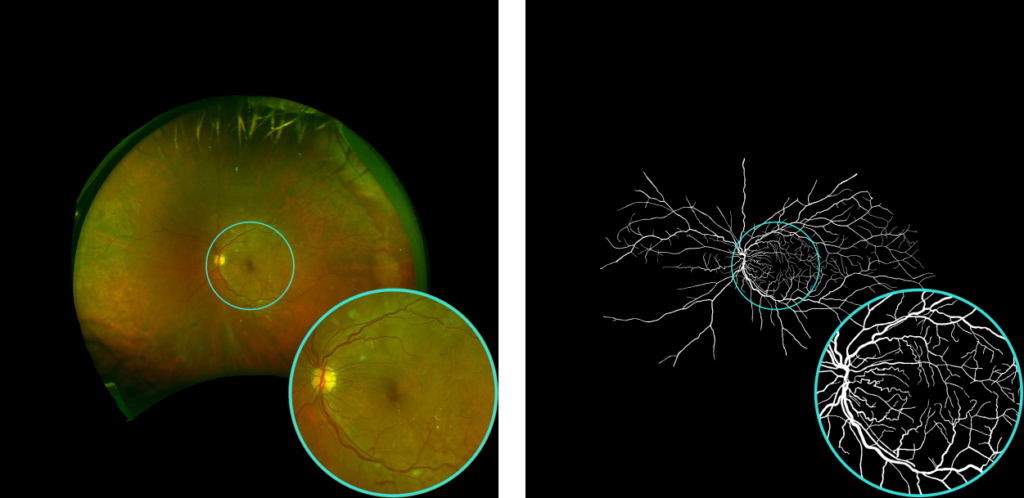
Example of labeled ground truth vessel map from the PRIME-FP20 dataset.
- Sample Results
- Quantitative results on PRIME-FP20 dataset
- Visual results on RECOVERY-FA19 dataset
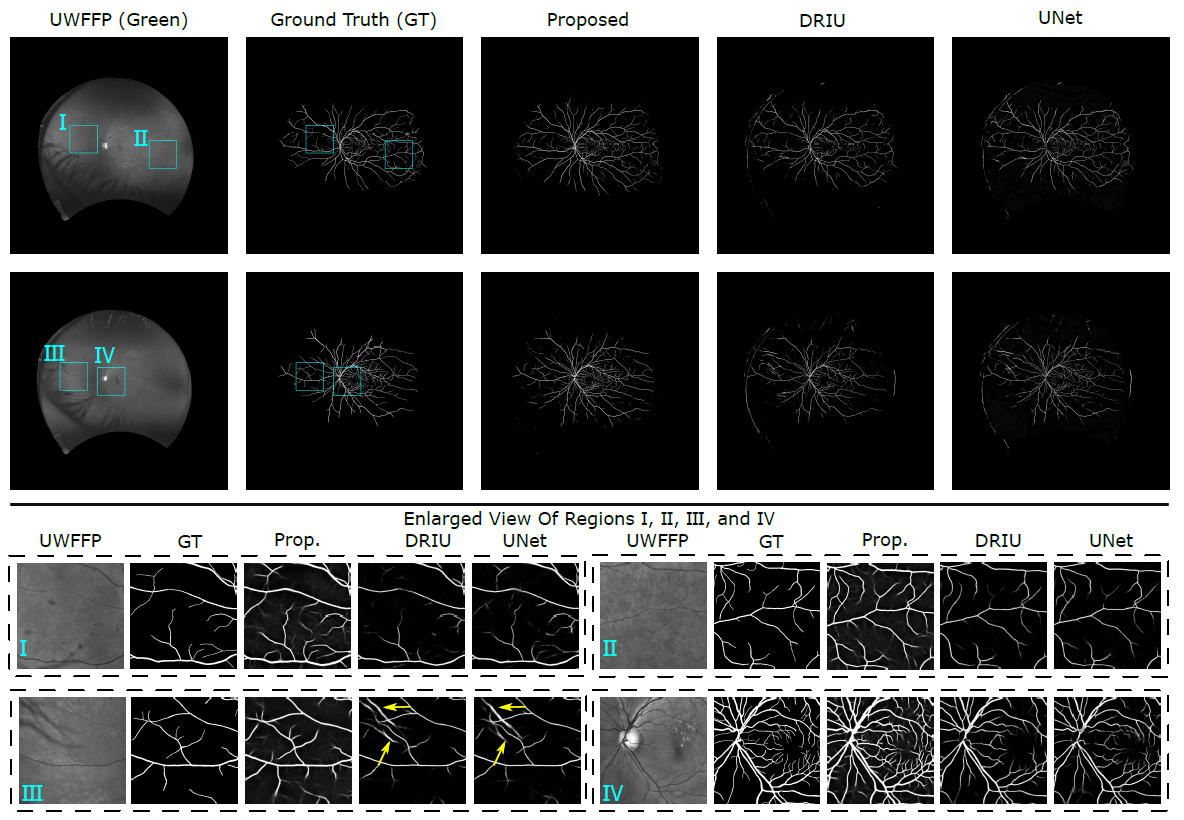
Visual comparison of results obtained with different algorithms for images from the PRIME-FP20 dataset. For each full image, two contrast-enhanced enlarged views of the selected regions (shown by cyan rectangles) are also included.
Publication
L. Ding, A. E. Kuriyan, R. S. Ramchandran, C. C. Wykoff, and G. Sharma, “Weakly-supervised vessel detection in ultra-widefield fundus photography via iterative multi-modal registration and learning,” IEEE Trans. Medical Imaging, accepted for publication, to appear.